In-Memory Multi-Valued Computing using Magneto-Electric Devices
Multi-valued computing can yield significant benefits compared to binary computing due to greater capacity and compressed encoding of information. Emergence of nanoscale devices that allow information encoding, computation and communication in multi-valued domain, provide an unexplored arena for efficient multi-valued logic (MVL) implementation. Moreover, it can provide unique opportunities for Non-Von Neumann computing and eliminating Von-Neumann memory bottleneck.
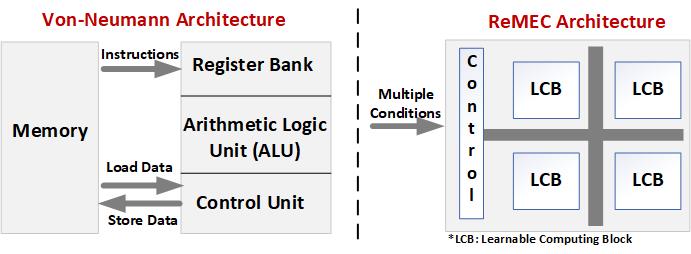
Non-Von Neumann Concept: Distributed learnable computing blocks that incorporates both memory and processing elements.
A key problem in moving towards MVL computing so far has been the complexity of MVL logic decomposition. Traditional approaches result in expressions that are very difficult to implement in hardware and ultimately yield little or no benefit when compared with Boolean. We are researching a new approach for MVL decomposition combining linear regression and pattern matching algorithms to obtain simplified linear expressions for any arbitrary MVL function. The linearization process can scale with circuit size and can be mapped to any technology.
For hardware implementation, native properties of emerging devices such can be used to map the linear equations and selecting conditions in hardware. Our technology independent hardware implementation of a quaternary adder revealed huge potentials for area, power and performance savings; for a quaternary adder, our results show at-least 62.5% saving in switching components vs. binary CMOS.
The new MVL decomposition and circuit implementation scheme combined with novel magneto-electric non-volatile devices can lead to Non-Von Neumann computing paradigm where computing elements can learn and can be reused for later usage without requiring memory access. The in-memory computing approach can reduce memory bottleneck and ultimately lead way for data mining, intelligent processing and neuromorphic processing.
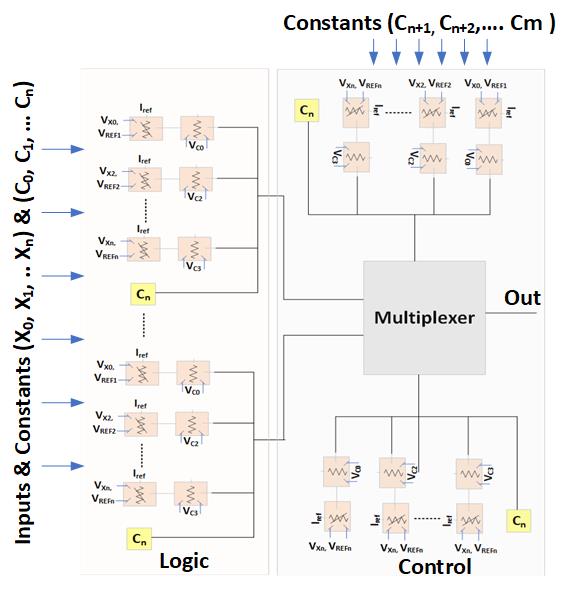